Weisong WEN (Chinese 文伟松)

Xiwei Bai, Weisong Wen, and Li-Ta Hsu. Using Sky-Pointing Fish-eye Camera and LiDAR to Aid GNSS Single Point Positioning in Urban Canyons, IET Intelligent Transport Systems, 2019, (SCI. 2019 IF. 2.74) [Submitted]
Abstract: Satisfactory accuracy (~2 meters) can be obtained in sparse areas. However, the GNSS positioning error can go up to even 100 meters in dense urban areas due to the multipath effects and none-line-of-sight (NLOS) receptions caused by reflection and blockage from buildings. NLOS is currently the dominant component degrading the performance of GNSS positioning. Recently, the camera is employed to detect the NLOS and then to exclude the NLOS measurements from GNSS calculation. The fully NLOS exclusion can cause severe distortion of satellite distribution, due to the excessive NLOS receptions in deep urban canyons. This paper proposes to correct the NLOS receptions with the aid of 3D LiDAR ranging after detecting NLOS receptions using a fish-eye camera. Finally, the GNSS positioning is improved using the healthy and corrected NLOS pseudorange measurements. The proposed method is evaluated through real road tests in typical highly urbanized canyons of Hong Kong. The evaluated results show that the proposed method can effectively improve the positioning performance.
Xiwei Bai, Weisong Wen, and Li-Ta Hsu. Collaborative Positioning based on Visual/Inertial with Multiple Vehicles.
Abstract: Making use of positioning information from multiple vehicles is a new way to enhance the positioning of single-agent vehicles. The data from multiple vehicles for collaborative mapping is also a promising application. In this research, we demonstrate the possibility of using positioning information from multiple vehicles to enhance the accuracy of single-agent. The cooperative positioning with multiple vehicles is tested in Whampoa of Hong Kong. Multiple vehicles are correlated based on loop closure. We collect the data using the same vehicle 4 times and postprocessing the data accordingly. This is prior research to validate the feasibility.
Weisong Wen, Di Wang, Yinghan Jin, Yiyang Zhou, Wei Zhan, and Li-Ta Hsu. High definition map-based localization for the autonomous driving vehicles.
Abstract: This research demonstrates the high definition map-based localization which is one of the most promising solutions. First, the high definition maps are generated which include the lane lines, building models, point clouds maps, etc. Then the high definition map-based localization is conducted accordingly. This work is finished during my visiting MSC Lab at UC Berkeley. During the point cloud map generation, the common pose graph optimization is applied to integrate the information from GNSS and LiDAR odometry. Moreover, the loop closure constraint is applied.
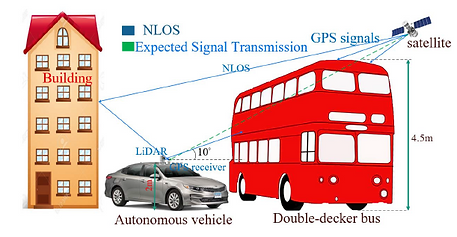

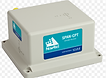

Weisong Wen, Guohao Zhang and Li-Ta Hsu, GNSS NLOS Exclusion Based on Dynamic Object Detection Using LiDAR Point Cloud, IEEE Transactions on Intelligent Transportation Systems, 2019, (SCI. 2019 IF. 5.744, Ranking 7.7%) [Minor revision and Resubmitted]
Abstract— Global Navigation Satellites System (GNSS) receiver provides absolute localization for it. GNSS solution can provide satisfactory positioning in open or sub-urban areas, however, its performance suffered in the super-urbanized area due to the phenomenon which is well-known as multipath effects and NLOS receptions. The same phenomenon caused by moving objects in the urban area is currently not modeled in the 3D geographic information system (GIS). Moving objects with a tall height, such as the double-decker bus, can also cause NLOS receptions because of the blockage of GNSS signals by the surface of objects. Therefore, we present a novel method to exclude the NLOS receptions caused by the double-decker bus in the highly urbanized area, Hong Kong. Both the static and dynamic experiments are conducted. The experiments certify that the dynamic objects are actually a factor that degrades the performance of GNSS positioning in urban scenarios.
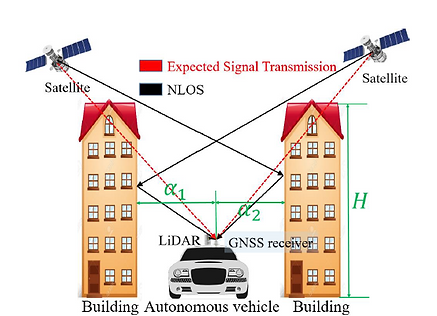

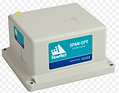

Weisong Wen, Guohao Zhang and Li-Ta Hsu, Correcting NLOS by 3D LiDAR and Building Height to Improve GNSS Single Point Positioning, The Journal of Navigation 61.1 (2019), (SCI. 2019 IF. 3.019, Ranking 10.7%) [Accepted]
Abstract—Occurrence of autonomous driving introduces high requirement in GNSS positioning performance. GNSS is currently the only source providing absolute positioning information. It is indispensable for initial position estimation for the high definition map-based localization solution in autonomous driving. Satisfactory positioning accuracy can be obtained in open space or sub-urban areas. However, its performance is heavily challenged in super-urbanized scenarios with the positioning error going up to even 100 meters, due to the well-known NLOS receptions which dominates the GNSS positioning errors. The recent state-of-art range-based 3D map aided GNSS (3DMA GNSS) can mitigate most of the NLOS receptions. However, ray-tracing simulation is time-consuming. Therefore, we present a novel method to detect the NLOS caused by surrounding buildings and correct the pseudorange measurements using 3D point clouds and building height without ray-tracing simulation. Extensive experiments are conducted to validate the proposed method. The results show that the proposed method can effectively improve the GNSS positioning.


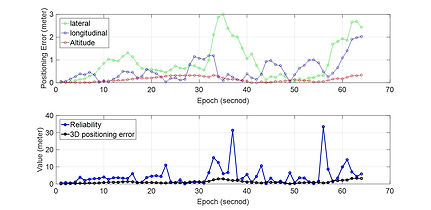
Wen, Weisong, Li-Ta Hsu, and Guohao Zhang. "Performance analysis of NDT-based graph SLAM for autonomous vehicle in diverse typical driving scenarios of Hong Kong." Sensors 18.11 (2018): 3928. (SCI. 2018 IF. 3.031)
Abstract—Localization is a significant part of an autonomous vehicle that needs robust and lane-level positioning. As an irreplaceable sensor, LiDAR can provide continuous and high-frequency six-dimension (6D) positioning by means of mapping, in the condition that enough environment features are available. In diverse urban scenarios, the environment feature availability relies heavily on the traffic (moving and static objects) and degree of urbanization. Common LiDAR-based localization demonstrations tend to be studied in light traffic and less urbanized areas. However, LiDAR-based positioning can be severely challenged in deep urbanized areas, such as Hong Kong, Tokyo, and New York with dense traffic and tall buildings. This paper proposes to analyze the performance of LiDAR-based positioning and its covariance estimation in diverse urban scenarios to further evaluate the relationship, between the performance of LiDAR-based positioning and scenarios conditions.
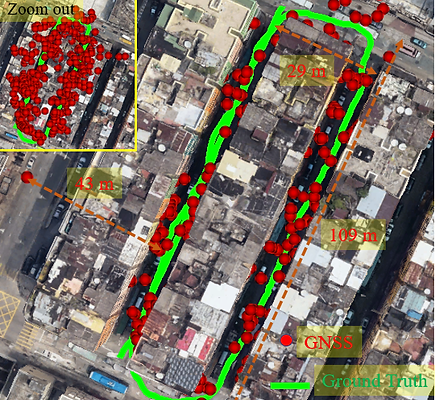
Weisong Wen, Guohao Zhang and Li-Ta Hsu, Object Detection Aided GNSS and Its Integration with LiDAR in Highly Urbanized Areas, IEEE Intelligent Transportation Systems Magazine, 2019, (SCI. 2016 IF. 3.294, Ranking 10.7%) [Accepted]
Abstract—Positioning is a key function for autonomous vehicles that require globally referenced localization information. LiDAR-based mapping, which refers to the simultaneous localization and mapping (SLAM), provides continuous and accurate positioning in the scenarios. However, the error of SLAM will accumulate over time. Besides, only relative positioning is provided by SLAM. Global navigation satellites system (GNSS) receiver is the only sensor to provide globally referenced localization and is usually integrated with the LiDAR in autonomous driving. However, the performance of GNSS is severely challenged due to the reflection and blockage of the buildings in super-urbanized cities, including Hong Kong and Tokyo and New York, causing the notorious non-line-of-sight (NLOS) reception. Moreover, the uncertainty of GNSS positioning is ambiguous resulting incorrect tuning during the GNSS/LiDAR integration. Innovatively, this paper employs a LiDAR to identify the NLOS measurement of GNSS receiver using the point-cloud based object detection. The experiment shows that the proper GNSS positioning error covariance is significant for GNSS/LiDAR integration.
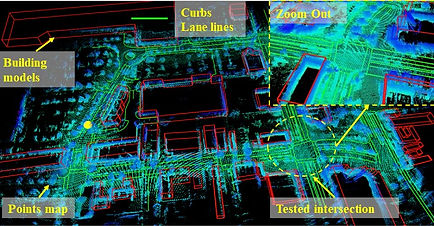


Wen, Weisong, Xiwei Bai, Wei Zhan, Masayoshi Tomizuka, and Li-Ta Hsu. "Uncertainty estimation of LiDAR matching aided by dynamic vehicle detection and high definition map." Electronics Letters (SCI. 2017 IF. 1.232).
Abstract—LiDAR matching between real-time point clouds and pre-built points map is a popular approach to provide accurate localization service for autonomous vehicles (AV). However, the performance is severely deteriorated in dense traffic scenes. Unavoidably, dynamic vehicles introduce additional uncertainty to the matching result. The main cause is that the pre-built map can be blocked by the surrounding dynamic vehicles from the view of LiDAR of ego vehicle. A novel uncertainty of LiDAR matching (ULM) estimation method aided by the dynamic vehicle (DV) detection and high definition map is proposed in this Letter. Comparing to the conventional Hessian matrix-based ULM estimation approach, the proposed method innovatively estimates the ULM by modeling surrounding DV. Then we propose to correlate the ULM with the detected DV and convergence feature of matching algorithm. From the evaluated real-data in an intersection area with dense traffic, the proposed method has exhibited the feasibility of estimating the ULM accurately.

Wen Weisong., Bai Xiwei., Kan Y.C., Hsu, L.T.* Tightly Coupled GNSS/INS Integration Via Factor Graph and Aided by Fish-eye Camera, IEEE Transactions on Vehicular Technology, 2019, (SCI. 2019 IF. 5.339, Ranking 7.7%) [Accepted]
Abstract—GNSS/INS integrated solution has been extensively studied over the past decades. However, its performance relies heavily on environmental conditions and sensor costs. The GNSS positioning can obtain satisfactory performance in the open area. Unfortunately, its accuracy can be severely degraded in a highly urbanized area, due to the notorious multipath effects and none-line-of-sight (NLOS) receptions. As a result, excessive GNSS outliers occur, which causes a huge error in GNSS/INS integration. This paper proposes to apply a fish-eye camera to capture the sky view image to further classify the NLOS and line-of-sight (LOS) measurements. In addition, the raw INS and GNSS measurements are tightly integrated using a state-of-the-art probabilistic factor graph model. Instead of excluding the NLOS receptions, this paper makes use of both the NLOS and LOS measurements by treating them with different weightings. Experiments conducted in typical urban canyons of Hong Kong showed that the proposed method could effectively mitigate the effects of GNSS outliers, and an improved accuracy of GNSS/INS integration was obtained, when compared with the conventional GNSS/INS integration.
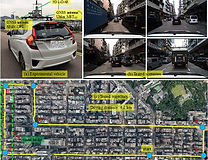

Wen Weisong., Bai Xiwei., Kan Y.C., Hsu, L.T.* AGPC-SLAM: Absolute Ground Plane Constrained Low-drift and Real-time 3D LiDAR SLAM in Dynamic Urban Scenarios, IEEE Transactions on Vehicular Technology, 2019, (Currently being submitted to ICRA 2020, to be submitted to the journal in the future)
Abstract—Accurate and robust positioning is critical for autonomous driving vehicles. 3D LiDAR-based simultaneous localization and mapping (SLAM) is a well-recognized solution. The state-of-the-art LiDAR odometry and mapping (LOAM) algorithm suffer from drifting, especially in vertical-axis and pitch angle. This drifting is serious when the vehicle is driving in urban environments with excessive dynamic objects. This is due to the fact that the 3D LiDAR has a very limited field of view (FOV) vertically and the surrounding dynamic objects can severely degrade the positional constraints in the z-axis. This paper proposes to detect the absolute ground plane to refine the state estimation of the vehicle. Different from the conventional relative plane constraint, this paper employs the absolute plane distance to refine the state estimation in the z-axis, and norm vector of the ground plan to constrain the rotation drift of LOAM. Finally, relative positioning from LOAM, constraint from absolute detection and loop closure are integrated under a proposed factor graph-based 3D LiDAR SLAM framework (AGPC-SLAM). We compared the proposed method with the existing state-of-the-art methods using real data collected in challenging dynamic urban scenarios (with numerous dynamic vehicles) of Hong Kong. The results show that the proposed method can obtain a significantly improved accuracy (2.73 meters of root mean square error (RMSE) in 4.2km driving distance) with real-time performance.